Demo Spaceman Slot
Demo Spaceman Slot: Main Demo Spaceman Pragmatic | Daftar Slot Pragmatic Spaceman Via Dana
Demo Spaceman Slot: Main Demo Spaceman Pragmatic | Daftar Slot Pragmatic Spaceman Via Dana
Couldn't load pickup availability
Demo Spaceman Slot: Main Demo Spaceman Pragmatic | Daftar Slot Pragmatic Spaceman Via Dana
Selamat datang di situs penyedia game pragmatic play tergacor paling sensasional di Indonesia. sebagai pecinta game demo slot online gacor sudah pasti mengenal permainan Slot spaceman demo yang paling fenomenal saat ini. tentunya didalam situs ini kalian akan menemukan berbagai kelebihan didalam akun demo slot spaceman serta memiliki tampilan tema yang sangat menarik untuk dimainkan sebab agar para slotmania tidak bosan memainkan seluruh game dari slot demo pragmatic terbaru. jadi sudah tidak diherankan jika akun demo spaceman pragmatic menjadi paling banyak dicari oleh kalangan pejudi remaja hingga dewasa. terlebih lagi demo slot spaceman rupiah Indonesia sangat mudah untuk menemukan kemenangan jackpot maxwin setiap saat. Tetapi kami sarankan sebelum bermain slot online spaceman lebih baik anda mencoba demo slot spaceman terlebih dahulu untuk mengetahui seluruh fitur dan pola slot gacor spaceman hari ini. untuk menemukan fitur demo slot spaceman Indonesia kalian hanya perlu daftar slot pragmatic spaceman di halaman situs ini untuk mengetahui seluruh kelebihan didalam demo slot spaceman terbaru.
Jika para pemain ingin mencari Slot demo pragmatic terlengkap anti lag dan tanpa deposit kalian bisa langsung mengunjungi halaman situs kami. sehingga kalian bisa memainkan slot demo pragmatic spaceman kapan saja dan dimana saja tanpa halangan. Terlebih lagi main demo slot spacemandi situs kami sudah mensupport tampilan di smartphone sehingga lebih mudah untuk dimainkan. jadi slot gratis pragmatic play no deposit sangat cocok untuk slotmania yang masih baru ingin merasakan kegacoran dari spaceman slot demo serta tanpa takut kehabisan modal. Tentunya halaman ini sangat cocok untuk anda dan juga slotters bisa mendapatkan pola slot spaceman yang bisa dimanfaatkan secara berulang kali.
Main Demo Slot Spaceman Dengan Link Slot Gacor Pragmatic Play
Main demo slot spaceman bisa kalian temukan didalam situs penyedia link slot gacor pragmatic play. Karena situs slot demo pragmatic play no deposit memberikan banyak jenis permainan slot online terlengkap termasuk slot demo spaceman pragmatic. Jadi kalian bisa menyaksikan ratusan game demo slot pragmatic maxwin no deposit gratis setiap hari dan temukan maxwin secara mudah. namun mencari link slot gacor hari ini pragmatic play tidak dapat dilakukan dengan mudah karena dijaman sekarang ini banyak situs penyedia akun slot demo pragmatic play tanpa deposit terbaru yang tidak sportif dan tidak bertanggung jawab. selain itu juga sangat jarang yang memberikan secara gratis atau secara Cuma Cuma. Jadi kami sebagai penyedia game demo slot spaceman gratis akan memberikan pengalaman yang sangat seru dan juga kalian akan menemukan pola slot spaceman yang sangat membantu anda dalam mendapatkan jackpot setiap hari.
Link slot gacor pragmatic play merupakan link yang paling dicari oleh pecinta game slot demo pragmatic play no deposit. karena kalian akan mendapatkan layanan dan keseruan yang tidak terdapat pada provider lainnya. seperti didalam game demo slot spaceman rupiah yang memiliki banyak fitur yang mendekatkan anda dengan maxwin. jadi pemain dapat merasakan jackpot maxwin disetiap waktu tanpa takut uang habis serta bisa menganalisa seluruh struktur pola slot spaceman secara berulang kali setiap hari.
Beberapa Keunggulan Yang Wajib Diketahui Oleh Pemain Akun Demo Slot Spaceman
Dengan mmemiliki Akun demo slot spaceman tentunya kalian akan mendapatkan beberapa keunggulan yang harus diketahui oleh seluruh petaruh demo slot spaceman pragmatic play. Terlebih lagi kalian dapat menemukan akun demo spaceman rupiah di situs penyedia link slot gacor pragmatic play kami dengan mudah. tentunya kalian akan mendapatkan beberapa keunggulan main spaceman slot demo seperti:
- 1. Tampilan spaceman demo slot yang lebih menarik
- 2. Memiliki dukungan dari provider pragmatic play gacor
- 3. Hanya dengan modal kecil bisa mendapatkan maxwin yang besar
- 4. Dapat dimainkan dengan perangkat smartphone
Spaceman demo slot memberikan berbagai macam koleksi yang sangat menarik seperti tema tema yang tidak membosankan. Serta menyediakan ratusan pilihan game yang memiliki gaya bermain yang berbeda beda dan unik. Tidak hanya itu kualitas grafik dan audio yang sangat enak dipandang, serta menyediakan bonus dan hadiah jackpot yang besar juga.
Demo slot spaceman merupakan game yang dikembangkan oleh pragmatic play gacor. tentunya pragmatic play merupakan provider ternama di dunia yang mengeluarkan game tergacor saat ini. jadi game pada demo slot spaceman pragmatic play sudah pasti terpercaya dan aman untuk dimainkan.
Tentunya dengan modal kecil saja kalian sudah bisa mendapatkan maxwin yang besar didalam game slot demo spaceman rupiah Indonesia. hal ini tentunya membuat para petaruh sangat tertarik main demo slot spaceman dan mengincar spaceman demo slot rupiah.
Memainkan akun demo slot spaceman saat ini sudah bisa diakses melalui perangkat smartphone dan jaringan internet. Hal ini membuat para slotmania lebih gampang dalam memainkan game pragmatic play terbaru dimana saja dan kapan saja.
Cara Daftar & Login Slot Pragmatic Spaceman Dengan Mudah
Daftar slot pragmatic spaceman merupakan langkah awal yang harus dilakukan oleh pemain yang ingin mendapatkan akun demo spaceman pragmatic dan merasakan kegacoran dari game demo slot spaceman pragmatic play. Tentunya akun demo slot spaceman rupiah memiliki banyak keuntungan yang bisa kalian dapatkan seperti tersedianya pola slot gacor spaceman jadi kami akan memberitahu cara daftar dan login slot pragmatic spaceman secara cepat dan mudah:
- 1. Cari link slot demo spaceman terpercaya di google
- 2. Klik daftar yang sudah dilampirkan dihalaman tersebut
- 3. Masukan seluruh data diri dengan benar dan valid
- 4. Klik daftar jika sudah selesai
Jika sudah melakukan daftar demo slot spaceman gratis maka kalian akan langsung diarahkan ke halaman login dan slotmania masukan username dan password yang sudah kalian daftarkan sebelumnya. Cari game slot spaceman demo didalam provider pragmatic play lalu atur jumlah taruhan dengan sesuai modal anda. Setelah itu ikuti intruksi seluruh permainan dan nikmati seluruh keseruan bermain slot demo spaceman pragmatic.
Rasakan Kemudahan Bermain Demo Slot Pragmatic Spaceman Via Dana
Dijaman sekarang ini untuk melakukan deposit slot spaceman pragmatic play sudah bisa dilakukan dengan mudah karena saat ini provider pragmatic play sudah memasukan metode pembayaran via dana yang sangat menguntungkan bagi slotmania yang belum memiliki akun banking. Dengan adanya Demo slot pragmatic spaceman via dana kalian dapat berdeposit dengan modal receh dan sudah berkesempatan menemukan maxwin setiap hari. tentunya menemukan situs slot dana spaceman sudah dapat dilakukan dengan mudah di internet. Tetapi pemain demo slot spaceman terbaru harus berhati hati terhadap situs penyedia link slot demo spaceman karena banyak situs yang tidak bertanggung jawab. maka kami sarankan untuk menggunakan halaman situs kami penyedia slot demo spaceman rupiah Indonesia terpercaya memberikan jaminan berapapun kemenangan anda pasti akan dibayar secara penuh. Pastinya kalian bisa menemukan link slot gacor hari ini pragmatic play kami di google.
Slot Zeus Gacor
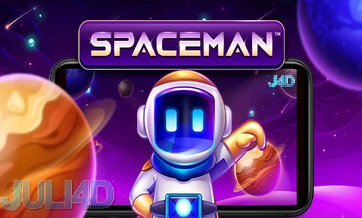